When a team of AidData researchers set out to study the impacts of Chinese development projects on ecologically sensitive areas, a common roadblock emerged: despite researchers’ best efforts, it’s not always possible to know exactly where aid is. While some of the available project records contained precise locations, many could only be traced back to the district or country level. And because China does not publicly disclose its development finance activities, better data was unlikely to be discovered. This led to a natural question: given this was the best data available, what meaningful questions could AidData researchers ask, and how should they do it?
Geospatial imprecision, or a lack of geographic coordinates for a given datapoint, impacts many geospatial datasets available today. In some cases, it is even intentional; for example, to protect anonymity in census or survey datasets. However, this imprecision challenges the ability of researchers to identify just how aid—or any other spatial intervention—impacts its surroundings.
Barriers to geospatial data collection make perfectly precise geospatial data the exception to the rule of “noisy” data with mixed levels of precision. Certain types of data (e.g., budget records) are allocated over entire countries or provinces, not to discrete coordinates. Finding ways to live and work with noisy and imprecise data will strengthen the analytical toolkit and give researchers more options. But how do you perform geospatial analysis on data that wasn’t necessarily meant for it?
One promising way forward is GeoSIMEX, a new analytical model developed at AidData that directly accounts for geospatial imprecision, without making potentially false assumptions or wasting imprecise data. An application of GeoSIMEX to analyze the impact of Chinese aid on land cover was recently published in the journal Development Engineering.
What is GeoSIMEX?
GeoSIMEX is a geospatially-adapted simulation and extrapolation (SIMEX) model available as an R package through AidData’s GEO program. It helps research models establish a relationship between measurement error and covariate bias introduced by geospatial uncertainty—an approach that is particularly useful if an analyst or researcher wants to estimate the impact of an intervention. This helps research models to avoid making false predictions because they unintentionally ignored a hidden source of bias: geospatial uncertainty.
How does GeoSIMEX help researchers deal with spatial imprecision?
GeoSIMEX establishes a trendline for measurement error due to spatial imprecision by simulating additional measurement error, and then extrapolating backwards to a theoretical condition of no error to get a range of possible values for the model’s predicted outcome.
Here’s a visual explanation of the technique, simplified from Figure 3 in the article in Development Engineering.
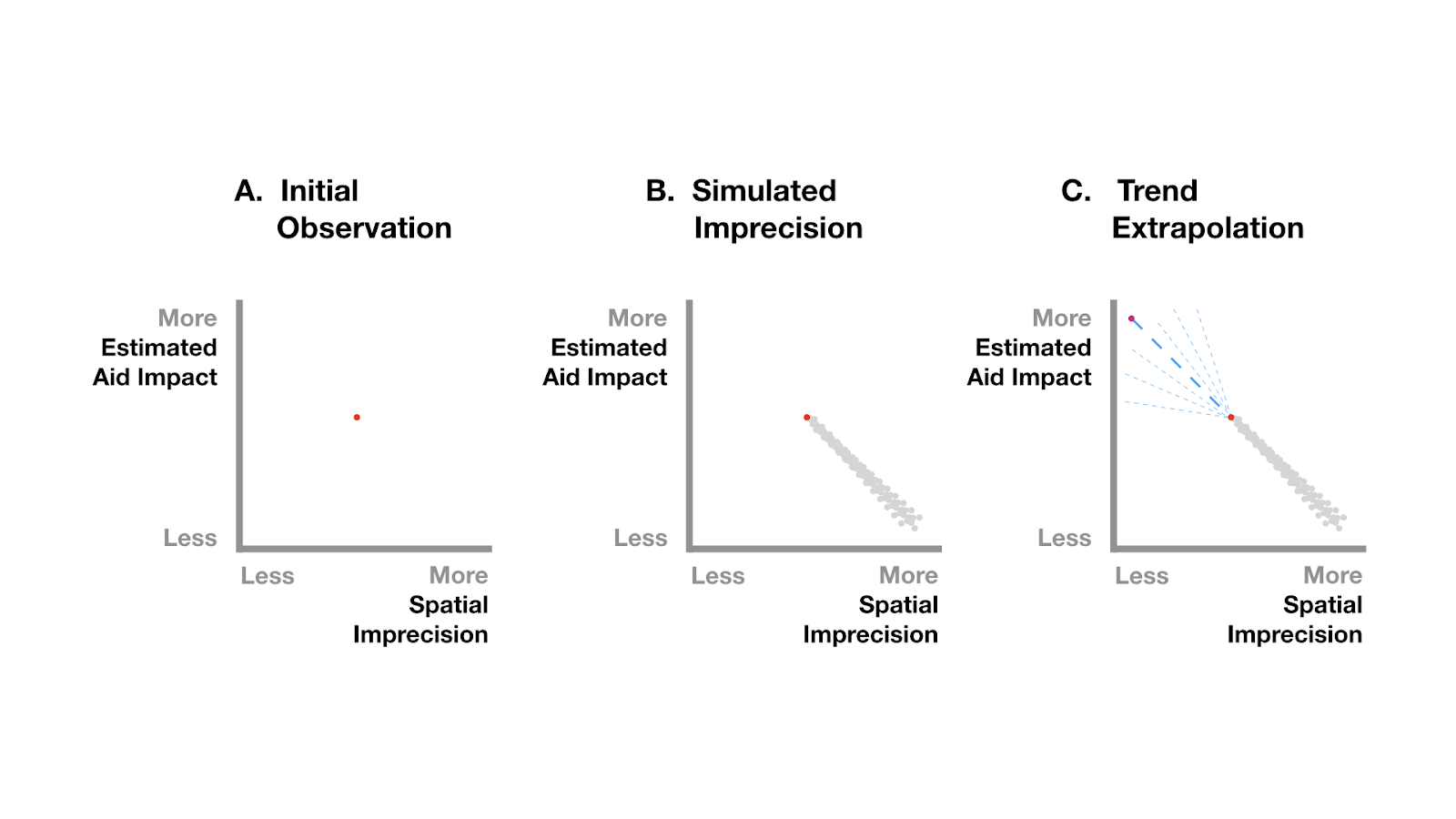
The figures above illustrate the relationship between the geographical precision of data and how strongly the model can explain reality. As spatial locations become less precise, the model gets noisier and it’s harder to see what’s going on. Conversely, more precise data means that the model is able to estimate what’s going on with more accuracy. By intentionally making a model noisier than it really is, we can start to understand the relationship between imprecision and the truth—essentially using increased imprecision to our advantage.
To do this, GeoSIMEX takes an initial snapshot of a relationship (for example, between aid and a change in poverty) and iterates through a thousand different simulations of what that relationship would look like with less geographically precise data (charts A and B above). By measuring how the model changes with decreasing geospatial precision, it generates a range of estimates for finer precisions than exist in the data (charts B and C above).
“What GeoSIMEX provides is a way to, with a few assumptions, explicitly model spatial imprecision and give you an idea of what the range—the true range—of errors are given the spatial imprecision in your data,” says Dan Runfola, AidData’s senior geospatial scientist and an author of the paper.
In practice, results of GeoSIMEX simulations will have a wide range of possible values. This has real implications for researchers: although GeoSIMEX presents a truer picture of reality, that picture ends up messier.
Previous workarounds to geospatial imprecision
GeoSIMEX is a promising way forward because it addresses two major trade-offs inherent to previous workarounds to geospatial imprecision: making potentially false assumptions and wasting imprecise data. Though GeoSIMEX is not the first workaround for handling geospatial imprecision, it has a number of advantages when compared to current practice.
One popular workaround is aggregating up to larger spatial areas. Imagine a dataset in which not every aid project will have precise geographic coordinates, but where there is enough information to tie every project to at least a region. Relying on national-level aggregates can obscure subnational trends and pockets of development activity, so researchers risk overlooking critical findings when they aggregate their data to higher geospatial levels.
In another popular work-around, researchers cherry-pick the data that has the most precision. When researchers only use data points with the highest spatial precision (typically only a small fraction of a dataset), they are letting a large portion of their data go to waste. In addition to the risk of inadvertently missing important trends hidden in less precise data, cherry-picking data also makes a critical assumption: that less precise data can be ignored safely without introducing bias. When this is not the case, cherry-picking only the most precise data increases the chances of research models suggesting false claims.
By directly accounting for the varying levels of geospatial imprecision that can exist in a dataset, GeoSIMEX allows researchers to use all of their data and avoid inadvertently introducing some of these biases into their analysis.
Moving forward with GeoSIMEX
GeoSIMEX is currently available as an R package on AidData’s GEO program page, so interested researchers can get the tool today. GEO also provides a link to GeoQuery, AidData’s one-stop shop for integrating spatial data on aid investments and outcome measures at fine geographic levels into a custom dataset. Researchers who are interested in learning more about how GeoSIMEX works can find a detailed outline of the analytic procedure in the associated paper in Development Engineering.
As more development organizations like KFW and the World Bank commit to using impact evaluations, it’s important that analysts and researchers find new ways to work with all of the geospatial data they have at hand—not just that of the highest precision. Being able to produce data of higher geospatial precision will prevent many headaches, but so will methods like GeoSIMEX that can more effectively analyze noisier and imprecise data.